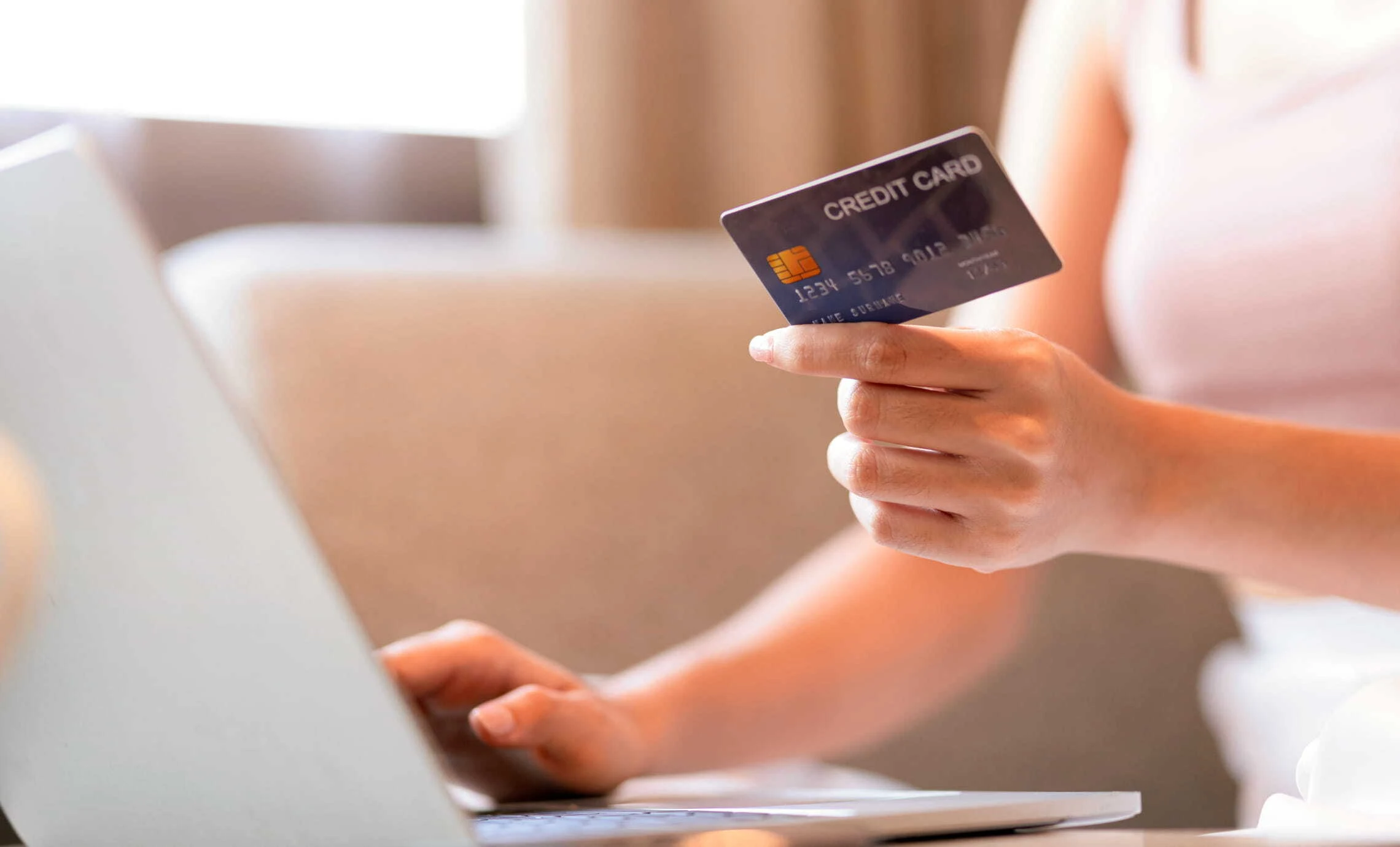
We publish the accuracy rates of our facial age estimation technology, split by gender, age and skin tone. By being open and transparent, we hope to give businesses and users confidence in the technology. We also hope this helps regulators to fairly review facial age estimation as an effective age assurance method.
However whilst evaluating facial age estimation, some stakeholders and regulators overlook the drawbacks and bias shown in other age assurance approaches.
Bias and limitations of using credit cards for age verification
One age check method approved in many countries is asking adults to use a credit card as evidence they are over 18. This is because credit cards (as opposed to debit cards) are usually only issued to those aged 18 or over. But this method is neither foolproof nor inclusive for everyone.
A child could use their parent or guardian’s credit card to pass an age check. There’s also the potential security risk of entering credit card details into different websites. And not everyone qualifies for a credit card which excludes them from this approach.
In the US, the Federal Reserve Board published a report looking at the economic well-being of households, including data on credit card access. 82% of adults in the US own a credit card. But this falls to 62% of younger adults, aged between 18 and 29.
The report concluded that credit card usage also differs by race, ethnicity and disability status.
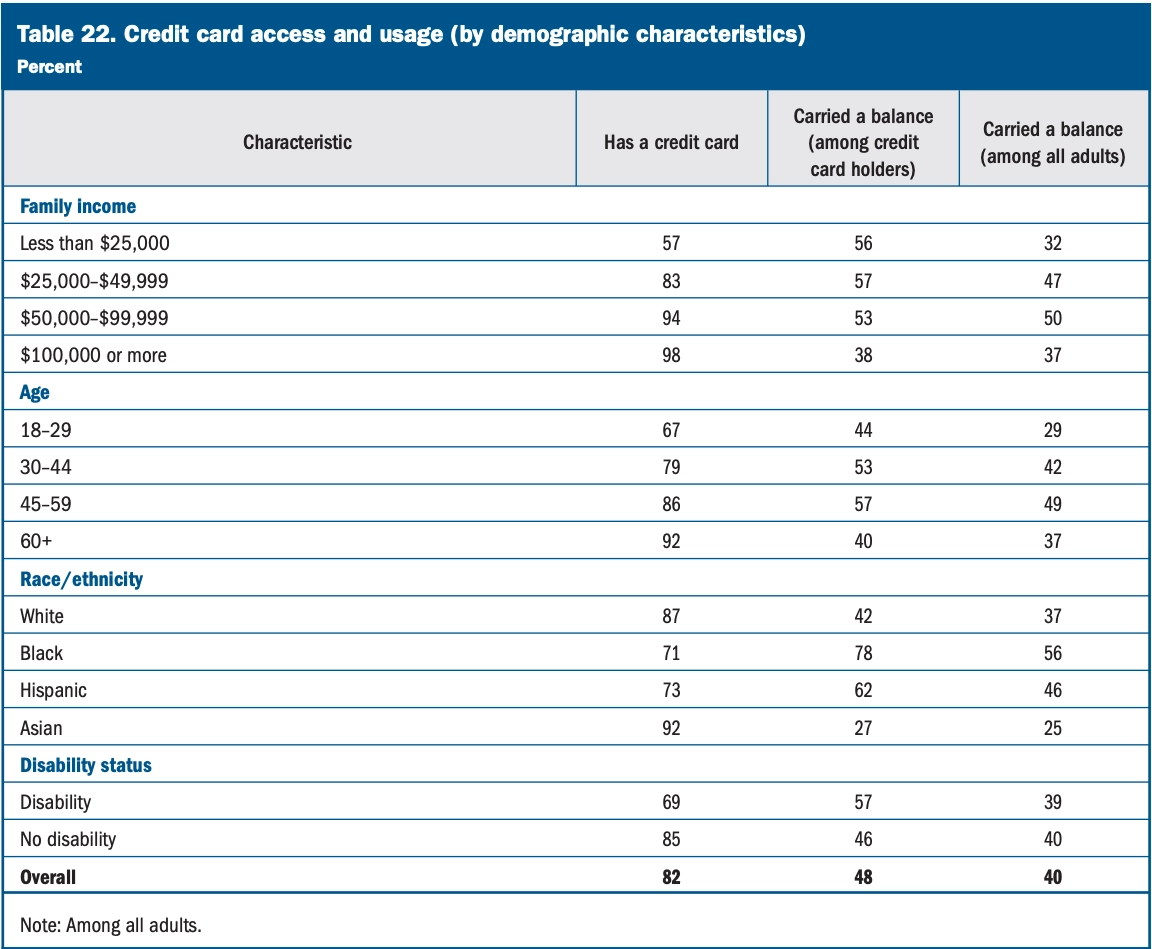
Income data from employment, savings and investments are the most influential factors in determining whether a credit card is issued or not. But it’s fair to say that more adults who are from certain racial or ethnic groups, or have a disability, face discrimination if a US regulation or organisation requires evidence of credit card ownership to access a service.
It’s also important to note that adults on lower incomes will find it harder to qualify for a credit card. These same adults may also struggle to buy other forms of identification, such as a passport, which can be expensive.
If companies rely on physical documents to access age-restricted content, goods or services, those from minority groups or those who are on lower incomes may lack the evidence required to prove their age.
Regulators considering age assurance will have to weigh up whether there are alternative methods for adults to choose from. This will be essential to making age checks effective, accessible and inclusive for everyone.
Yoti facial age estimation accuracy rates
Our latest facial age estimation results are fair to all children aged 13 to 17 when estimating whether these children are over or under 25. We correctly estimate 99.8% to 99.9% of 13 to 17-year-olds as being under 25 across the six skin tone groups.

Looking specifically at 17-year-olds being correctly estimated as under 25, the accuracy rates are consistent across different skin tones:

Yoti will always strive to reduce bias and be transparent with our results. We support regulators who want to be sure that age estimation does not discriminate against particular age groups, genders or skin tones.
But we know regulators have to make hard decisions on inclusion, fairness, choice and cost-effectiveness, to ensure age checking is inclusive and practical for individuals and businesses.
In the offline world, regulators rely upon humans to estimate the ages of customers. Whether it’s a retail worker at a supermarket or a security guard at a nightclub, humans need to estimate the age of individuals. This is to determine if the person in front of them is old enough to purchase an age-restricted item or access an age-gated service or experience.
Unfortunately, humans aren’t great at estimating ages. People often estimate age more accurately when assessing faces within their own age range. But younger people tend to overestimate the age of older faces and older people tend to overestimate the age of younger faces.
A study in this area reported a mean absolute error in human guesses of 4.7 years across an age range of 0 to 70. Across a range of people aged between 16 and 70, this rose to 7.4 years. In comparison, Yoti’s latest age estimation algorithm has a mean absolute error of 2.9 within this same age range.
People are also less accurate at estimating the age of individuals from other racial groups. And our ability to estimate accurately tends to decrease as we ourselves get older. People can also show personal favour or bias, even if this isn’t deliberate.
Despite this, regulators have decided that humans are sufficient at estimating the age of customers. They rely on this method to protect children by ensuring they can’t access age-restricted goods and services. It’s impractical to test the accuracy of every employee who has to estimate the age of customers – this could be supermarket workers, security staff or bartenders.
In comparison, facial age estimation technology and its accuracy levels can be measured, improved and reported on.
Facial age estimation independently tested for bias
The US National Institute of Standards and Technology (NIST) has just started testing performance results for facial age estimation algorithms. The first results should be published in November this year.
This is a crucial milestone. Until now, facial age estimation suppliers have mostly been ‘marking their own homework’. This does not allow regulators or the general public to completely trust the results. NIST testing is likely to show that leading algorithms are effective at estimating age but unsurprisingly, no algorithm displays zero bias across age, gender or race.
However facial age estimation offers many benefits where other age assurance methods fall down:
- Free for users: facial age estimation is free for individuals to use
- Privacy-preserving: people do not need to enter any personal information or share sensitive data displayed on their identity documents. It is not facial recognition and the image used to estimate age is always deleted
- Inclusive: the technology estimates someone’s age from a facial image without the need for a physical identity document or credit card
Current age verification methods are not perfectly fair. Not everyone meets the criteria to get a credit card, some people can’t afford a passport, and others aren’t old enough to have a driving licence. Yet these are the methods regulators and businesses currently rely on.
For age checking to be inclusive for everyone, it’s vital that regulators weigh up the benefits of each age assurance method. Adults should have a choice in how they prove their age. This ensures age checking does not exclude certain people, especially those who do not own physical documents.
If you have any questions about the accuracy of our facial age estimation technology, please get in touch.