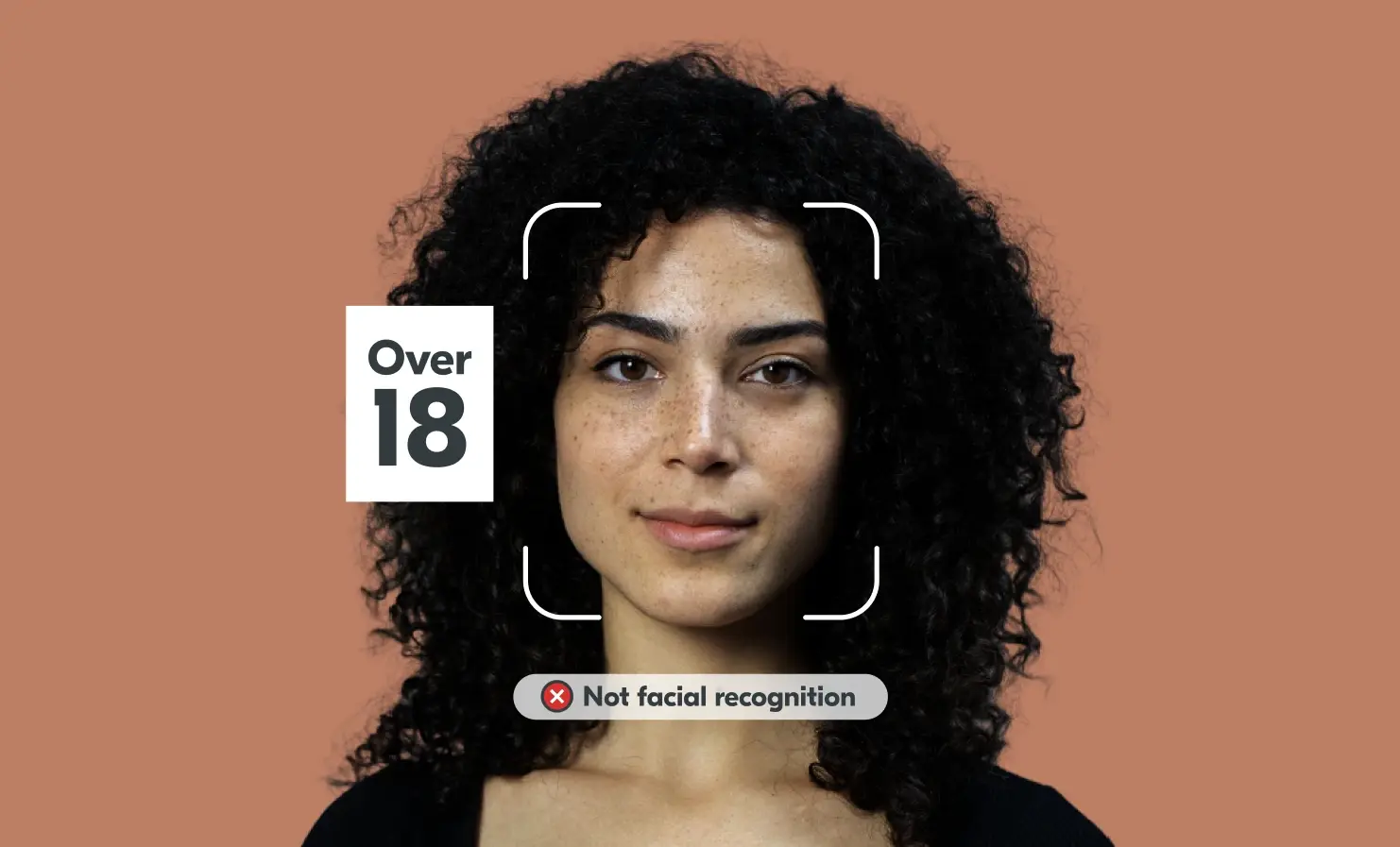
There’s quite a bit of confusion about the differences between facial age estimation and facial recognition. While both types of technology work with images of faces, they’re used for different reasons and are trained in different ways.
To help clear up some of these misconceptions, we’ve explained some of the key ways that our facial age estimation is not facial recognition.
Facial age estimation vs. facial recognition: designed to give two different outcomes.
Facial age estimation delivers an estimated age result. Facial recognition delivers a match (or no match) between images of a person.
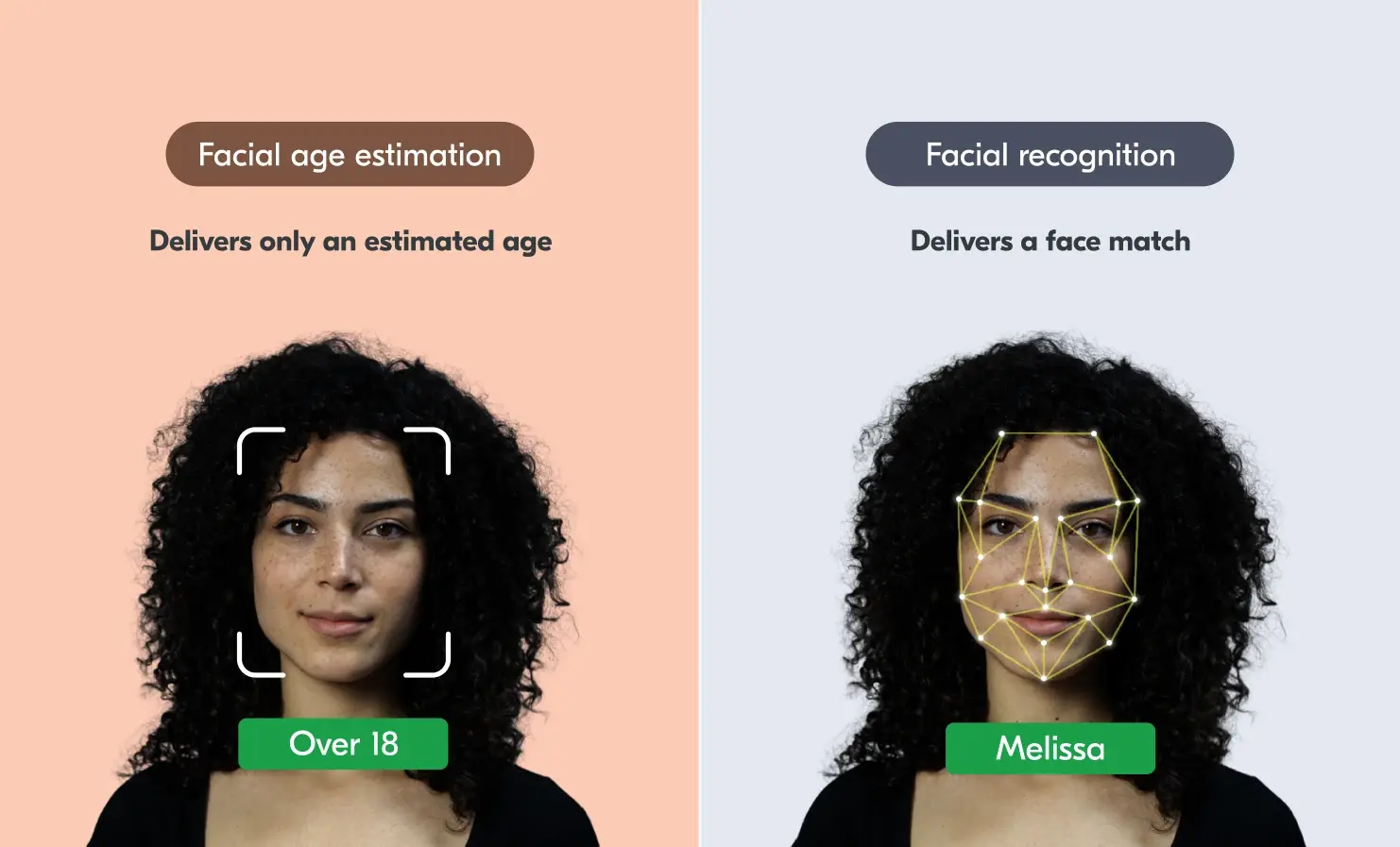
The objective of facial age estimation technology is completely different and separate from the objective of facial recognition.
In its simplest form, the purpose of facial recognition is to either identify or confirm a person’s identity from their face. Facial recognition is able to match one face (from either a digital image or video) with another face. This could be from a photo on an ID document or from images stored within a database.
With this in mind, facial recognition technology is built to deliver one of two results: a match or no match. If facial recognition is used to match a face to one in a database, it may pull up all the information stored within that database about the person it has recognised. This could range from personal information such as a name or date of birth, to browsing habits or social media accounts. Basically, whatever information has been previously inputted into that database.
On the other hand, the purpose of facial age estimation is to estimate a person’s age from a facial image. The result delivered by facial age estimation differs from facial recognition.
Instead of delivering a match or no match, facial age estimation technology is built to deliver a numerical value. Depending on regulatory requirements, these could be one of three results. If we take the example of a 15-year-old, these three results could be:
- 15 years old (i.e. an exact estimated age)
- between 13 and 17 years old (i.e. an estimated age range)
- over 13 or under 18 (i.e. an “over” or “under” age result)
Once this age result is delivered, this will indicate whether the person (whose identity is unknown) will be able to access the relevant age-restricted goods, services or experiences.
Facial age estimation is trained in a different way to facial recognition.
“Facial age estimation is trained on photos accompanied by known-age values. Facial recognition is trained on pairs of photographs with identity labels.” – NIST, 2024
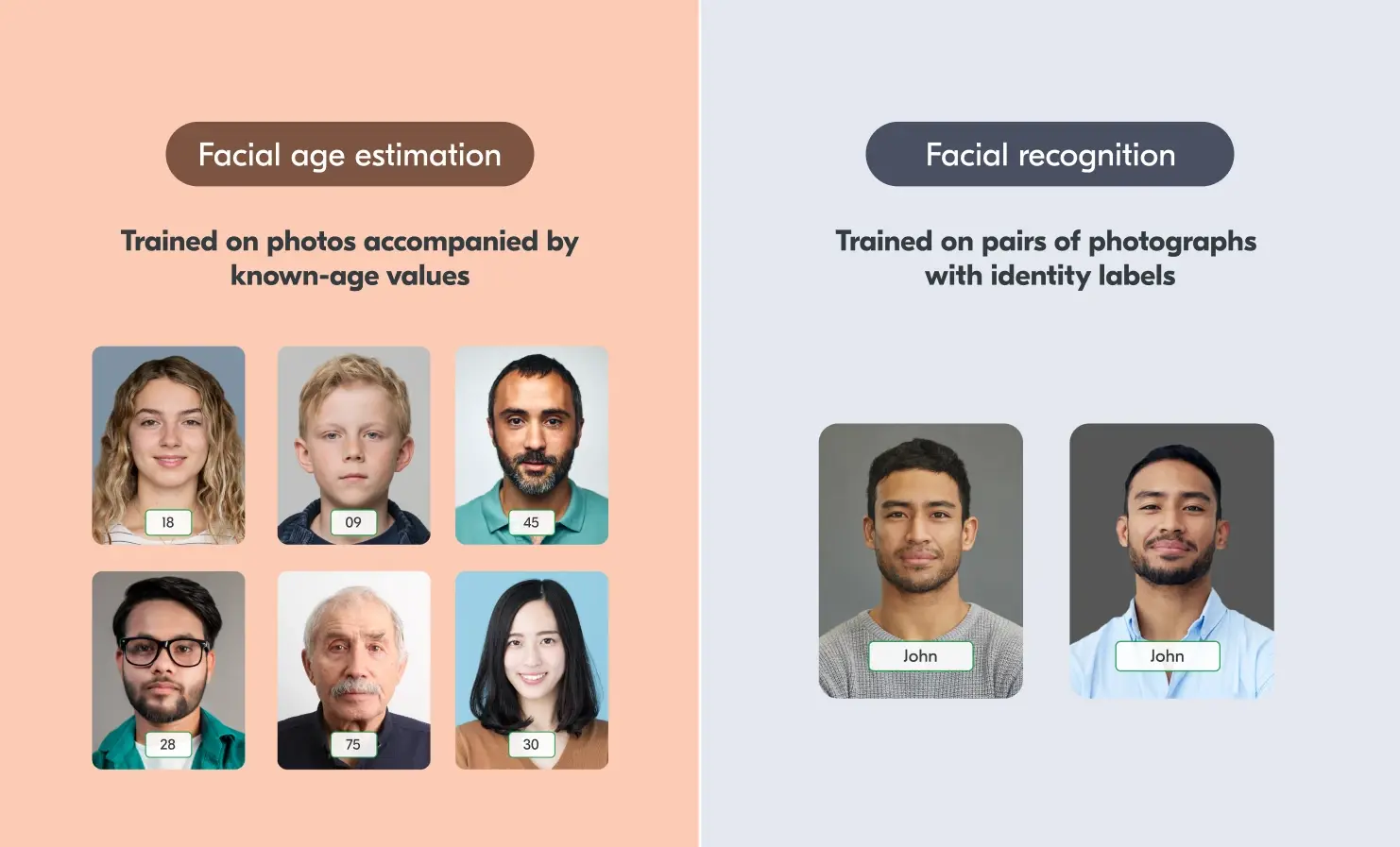
Facial age estimation and facial recognition both rely on a modern technique called “deep learning” and they both start with a face. But that’s where their similarities end. The two models are trained with entirely different objectives and on different data.
Our facial age estimation doesn’t have the ability to match or identify faces because it simply hasn’t been taught how to do so.
In fact, if a facial age estimation model was able to identify the people it had been trained on, it wouldn’t be suitable for the task of estimating age. This is because it would always return the same age for these people even as they continue to age. For example, if someone was 16 when the model was trained, it would always estimate that person as being 16. This wouldn’t be useful if that same person wanted to, for instance, buy age-restricted goods a few years later, and needed to prove that they were over 18.
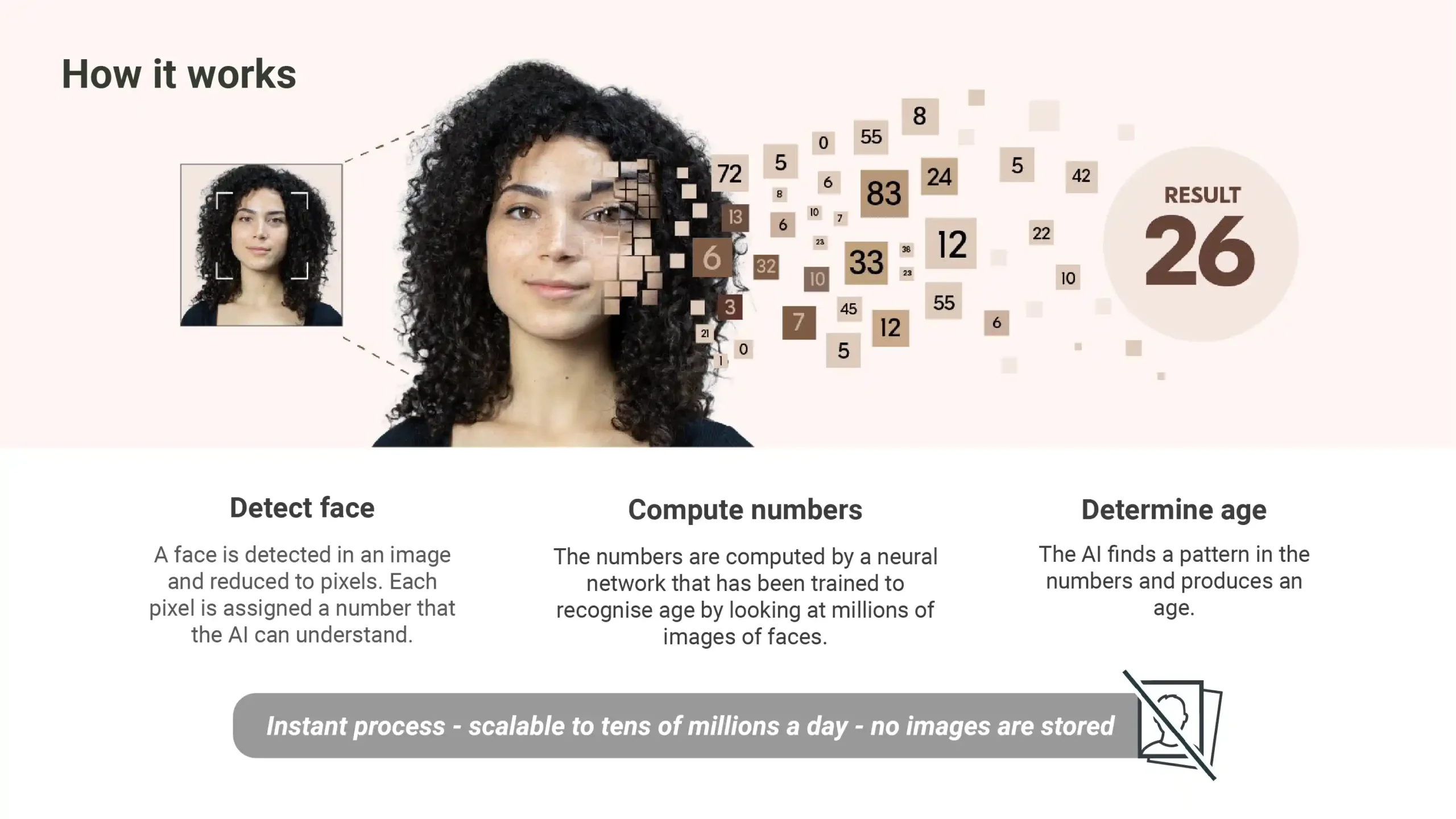
Facial age estimation is performed by a ‘neural network’. The algorithm has learned to estimate age in the same way humans do – by looking at faces.
It works by converting the pixels of a facial image into numbers and comparing the pattern of numbers to patterns associated with known ages. The technology detects a live human face, analyses the pixels in the image and gives an age estimate.
Facial recognition works in a different way to facial age estimation. The National Institute of Standards and Technology (NIST) states that facial recognition “compares identity information extracted from two photos with the goal of determining if they are from the same person. The first sample is stored in a passport, or phone, or database. The second sample is collected for verification or identification”. The purpose of facial recognition is to match the new facial image with one that has been previously captured, and therefore with all the data associated with that image.
Unlike facial recognition, facial age estimation technology is not trained to match an image of one face to the image of another. It simply analyses the pixels of the facial image, delivers the age result, such as ‘over 18’ and then deletes the image. It’s not able to identify people or recognise faces.
Facial recognition relies on existing images. Facial age estimation has no existing images.
For facial recognition to work, it needs to match a new face to an existing image. Facial age estimation cannot match faces as no images are saved or stored in the first place.
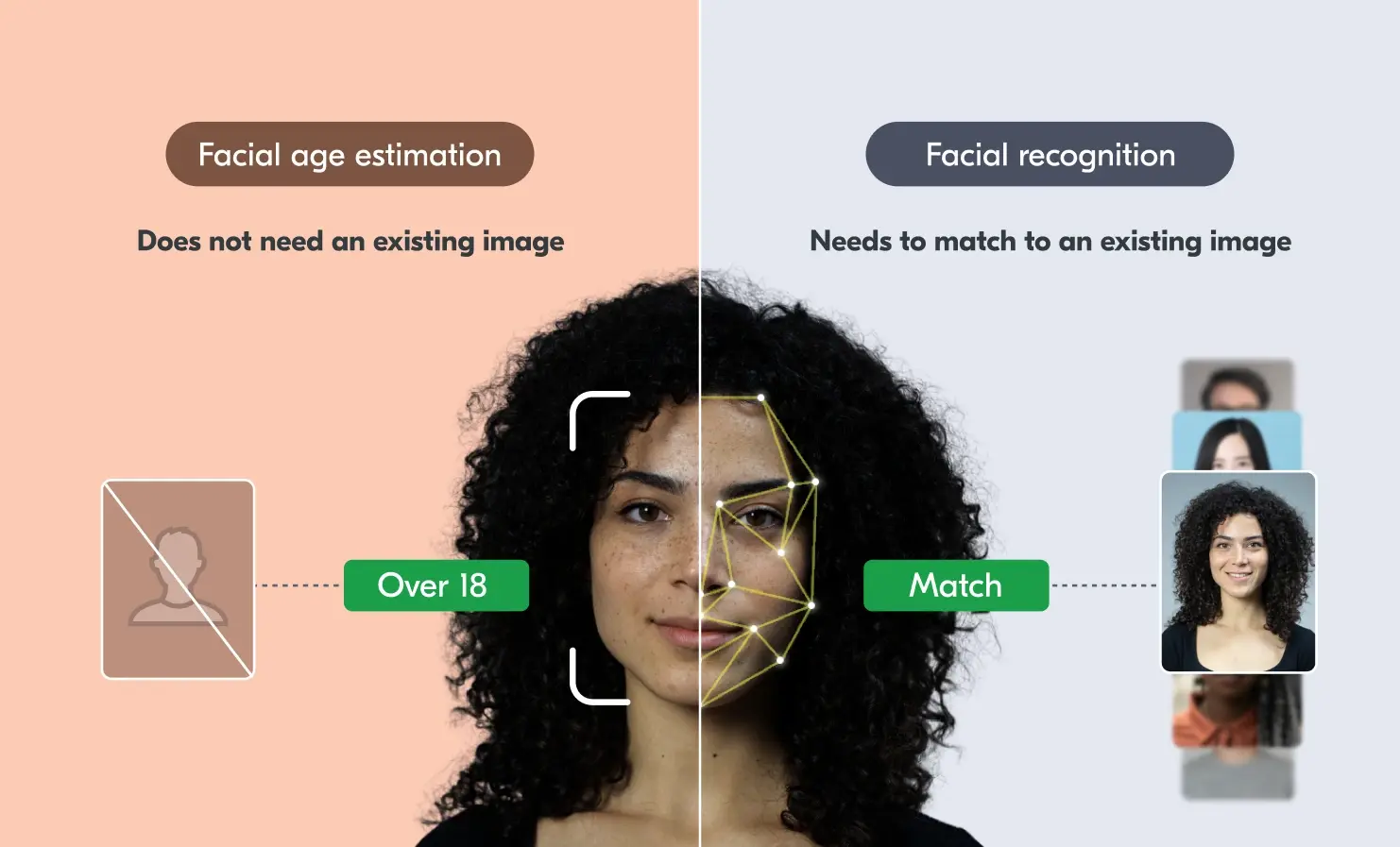
For facial recognition to take place, there needs to be at least one other facial image for the technology to match the original image to. This is so the system can compare the image of the face presented to it with all existing images. Otherwise, there’s no way of a person “being recognised”.
When facial age estimation is used in practice, facial recognition cannot take place as there’s no database to begin with. There’s nothing to compare the facial image to. This means that one face cannot be matched to another.
As soon as an age estimate is delivered, we permanently delete the facial images in real time. We do not use them for our own learning or training purposes. This is externally reviewed as part of our SOC 2 and PAS1296 assessments.
The images are not stored, shared, sold on or used for any other purpose. And since no data is saved, there’s no way for us to create a database of users.
During development, facial recognition models require different training images than facial age estimation models.
During the training and testing stages, facial recognition models require multiple facial images of each person, taken at different angles, with different cameras, in different environments. In contrast, facial age estimation models only require a single facial image of each person with a corresponding month and year of birth.
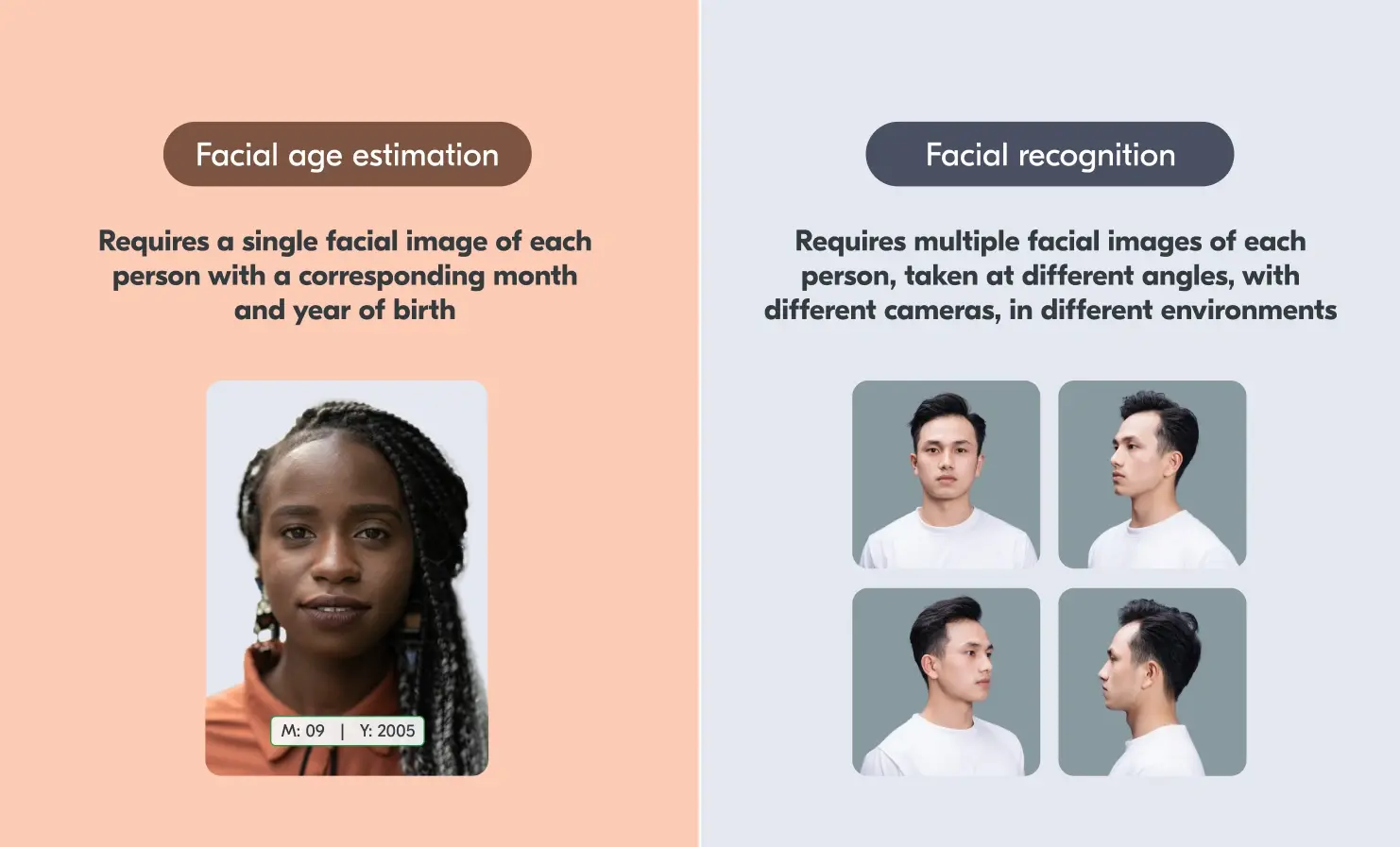
The first step in developing our facial age estimation model is what we call “the training stage”. This is when the algorithm is trained with accurate age data. The model is trained with lots of images, paired with their corresponding age values, until it arrives at a processing formula that works best.
At this stage, the only information we require is a single facial image and the corresponding month and year of birth (but not the exact day they were born).
After this, the second step is “the testing stage”. Here, the algorithm is tested on a new dataset to evaluate how well it performs. For this stage, the only information required is, again, a single facial image and the corresponding month and year of birth. We need to know how old the person in the photo is, so that we can check that the algorithm is correctly estimating their age.
Although facial recognition systems are trained in a similar way, the types of images required are vastly different.
Since the objective of facial recognition systems is to be able to match one face to another, it needs multiple images of the same person. These images should ideally be taken at different angles, with different cameras, in different environments. Preferably, it would also be trained on the same people over an extended period of time too. Otherwise, the model will only be able to recognise that person if they were in the same environment, position and lighting conditions as the original training image.
Therefore, even if an attempt was made to recognise a face using a facial age estimation model, it would perform terribly. This is because these two models are trained on different – and “orthogonal” – objectives. A facial recognition model is trained to consider images of the same person to be “similar”, regardless of their age. On the other hand, facial age estimation is trained to group together images of people of the same age, regardless of who they are.
Yoti’s facial age estimation is not facial recognition.
Our facial age estimation is not facial recognition as they’re designed to do fundamentally different things. Facial recognition systems are designed to match faces to other faces within an existing image. On the other hand, facial age estimation technology is trained to give an age estimate of the unknown face that is presented to it, and then delete the image.
As a result, facial recognition systems can’t estimate age, and similarly, our facial age estimation system can’t recognise faces.
If you’d like to take a deeper dive into exactly how our facial age estimation works, take a look at our latest white paper or get in touch.