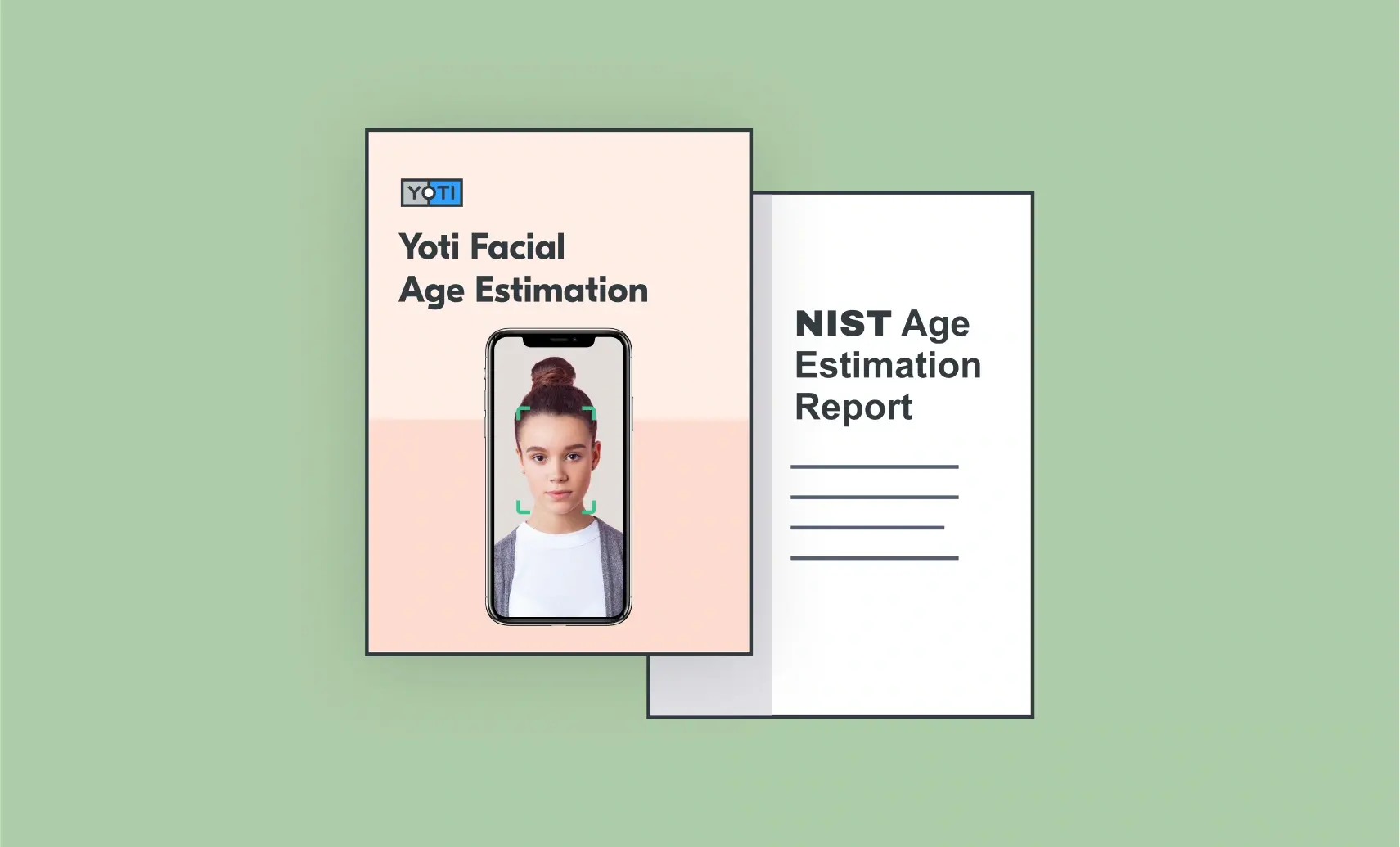
In September 2023, we submitted our facial age estimation model to the US National Institute of Standards and Technology (NIST), as part of a public testing process. This is the first time since 2014 that NIST has evaluated facial age estimation algorithms. NIST age estimation reports are likely to become a globally trusted performance guide for vendor models.
NIST assessed vendor Facial Age Estimation models using 4 data test sets at certain image sizes:
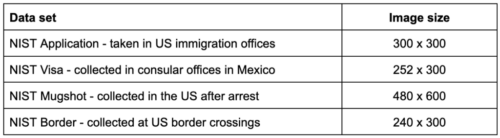
NIST provides some example images:
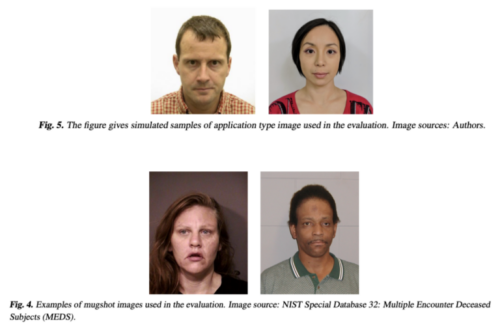
NIST note in their report that age estimation accuracy “will depend on the quality of the images” and the type of facial images captured.
For 6 years, Yoti have trained our model on primarily selfies of people looking into a mobile phone camera (or a laptop camera) because this is the obvious way customers can capture (live) their facial image to be age estimated. We capture these facial images at 720 x 800 pixels, with the face closely cropped to maximise the facial detail, because we have learned that we can attain higher age estimation accuracy for businesses by using this image size.
We believe our training and testing on mobile phone images with closely cropped faces at 720 x 800 image size are key reasons why Yoti published MAEs (and FPRs) are lower (more accurate) for the Yoti model than the performance data published by NIST their 4 different test data sets.
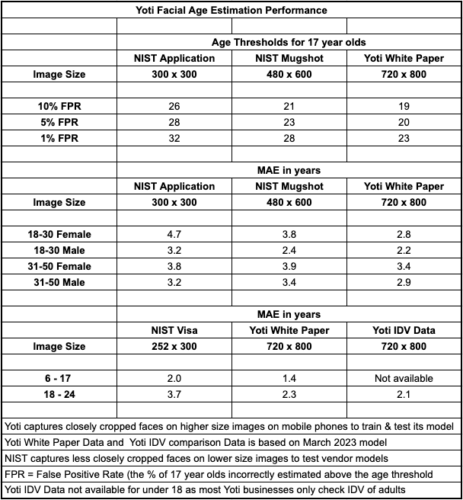
NIST selected FPR objectives of 10%, 5% and 1% in their report as a way to benchmark their evaluation. As can be seen from the table above, NIST publish that Yoti’s age estimation model is more accurate on higher image size ‘Mugshot’ faces than lower image size ‘Application’ faces. Consequently, the age thresholds required to meet FPRs of 10%, 5% and 1% are lower for Mugshot images than those needed using Application images. The age thresholds required to meet these FPR %s are lower still when the Yoti model is estimating age from mobile phone captured, higher image size, facial images.
NIST used over 11 million facial images (with verified age) to test vendors. Some readers may wonder why NIST did not also test vendors with a test set of mobile phone camera facial images given, this is how most images will be captured for online age estimation.
The reality is that it is very challenging to capture, with consent, a database of millions of mobile phone facial images with ground truth date of birth evidence from individuals representative of many countries across the world.
Yoti is fortunate to have a very large set of consented and anonymised facial images, verified to government issued age data, from Yoti app users. By separating out ~120,000 of these images as diverse test data across each year of age, from the many millions of images used to train our algorithm, we have confidence in the accuracy figures we publish in our white paper (based primarily on mobile phone facial images at 720 x 800 pixels).
As part of our document authenticity in our identity verification service we compare the age estimation result of the selfie with the real age from their document, which also helps us test the accuracy of the model.
Finally Yoti’s facial age estimation model was first tested for accuracy, and positively certified, in November 2020 by ACCS, a UK accredited testing agency. Our age estimation model is used by some of the largest online brands, including Meta and OnlyFans, both of whom have publicly stated that it works very well.