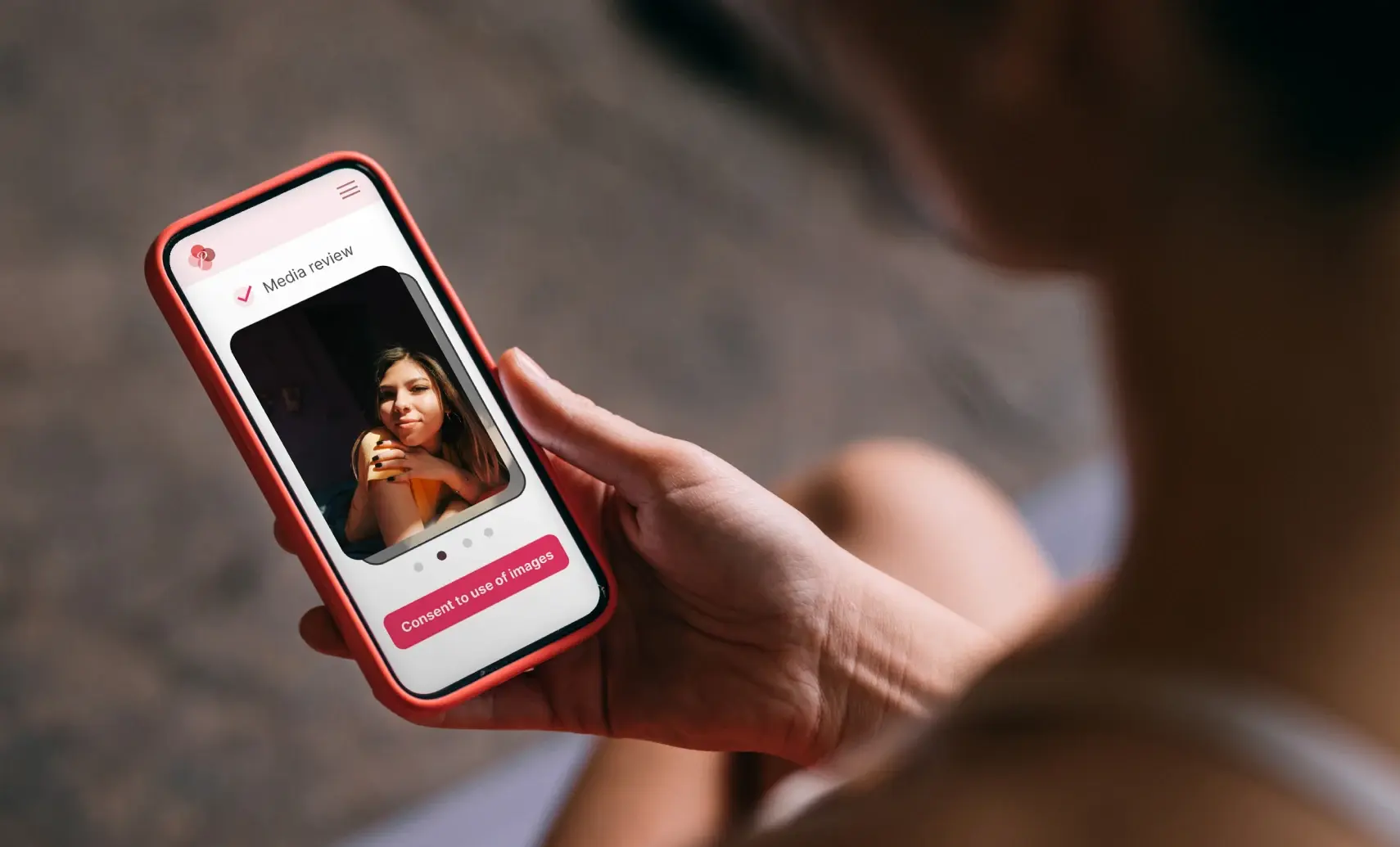
It’s concerning to see how innovative artificial intelligence (AI) is being used to create deepfakes that are spreading disingenuous information and explicit images online.
Deepfakes are realistic videos or images created by generative AI. Fraudsters can now use advanced algorithms to manipulate visual and audio elements that mimic real people. This fake content shows people doing or saying things they never did.
Two prominent individuals have recently been targets of deepfake scams. A video featuring Taylor Swift generated by artificial intelligence was used to promote a fraudulent cookware competition, and explicit AI-generated images of her were widely circulated online. Additionally, a voice recording pretending to be President Joe Biden was circulated to dissuade people from voting in the polls.
These incidents highlight the urgent need to address the threat of deepfakes. Bad actors are capitalising on the opportunity to inject disingenuous information online. As AI technology continues to develop at an astonishing rate, these threats will only get worse.
One of the issues with deepfakes, particularly images, is that once they are out in the wild, they are very difficult to detect. Videos and voice notes can, at the moment and in some cases, be spotted due to the temporal flow of the media. However, given the accelerated rate of improvement in these models, they will also be very challenging to detect once online.
Identifying fake images is becoming increasingly challenging for even human moderation teams. This causes significant mental stress and puts pressure on the moderation teams. However, existing tools can help mitigate this problem, increase efficiency, and reduce platform costs.
We aren’t suggesting we have the whole solution, it would require a collaborative effort across different parties.
- Platforms – will need to implement further policies – not necessarily ruinist, but that would drive away or suppress bad actors whilst simultaneously increasing trust, with very little effect on friction
- Video and image creation tools – efforts are also underway by image and video production tools to add digital watermarks to content. This will obviously help authentic content, but still the issue remains for inauthentic content.
- Creators – buying in to new processes to ensure their intellectual property or indeed image is not compromised.
How Yoti can help to combat deepfakes
The key to combating fake images is to use technology to detect and prevent them from being uploaded. If you only look to tackle the issue once content is live, it’s too late.
Let’s look at three examples:
- Intimate images
- High-profile celebrities and public figures
- Verification and authentication for account holders
Intimate images
There are multiple companies that are able to detect if an image or video contains nudity. This can be automatically flagged and trigger a requirement to gain consent from the account owner to ensure they are the person in the image. This is the part Yoti can help with. We provide technology to match the person uploading the content with the image – ensuring it is the same person in the intimate image. We can then capture consent for all participants in a particular image or video.
In genuine cases, consent can be easily provided using our trust and safety tools. Where it’s a deepfake image, the individual would not provide their consent. This would prevent the spread of misinformation and non-consensual, intimate imagery of real people.
Any suspicious content can be stopped at source. This would automate the process, tackling deepfakes at scale and reducing pressure on human moderation teams.
High profile celebrities and public figures
For celebrities and well known public figures, their profile can be added to a ‘watch list’. Any content uploaded which features their image can be immediately flagged as potentially problematic, requiring a further check.
This can work in many ways:
- Yes, this person has consented – no further checks are needed
- Yes, the content is from their verified profile – no further checks are needed
- We don’t recognise this person – need to get consent before the content is uploaded
- No, this person is banned from the platform – the content needs to be removed
- No, this person is potentially underage – the content needs to be removed
By verifying content upon upload, deepfake images and videos can be prevented from appearing online. These checks can also be completed during live streaming – ensuring there is a real person in the content. Our anti-spoofing liveness technology, evaluated by iBeta to the National Institute of Standards and Technology (NIST), is an essential tool to protect people from deepfakes.
This could easily extend to a wider audience too. Members of the wider population could ‘opt-in’ to a service that would ensure no graphic or AI-generated content could be submitted to online platforms that participated.
This could all be achieved using a 1:N or 1:many facial recognition product such as Yoti MyFace Match.
Verification and authentication for account holders
Another emerging issue is deepfakes being used for high-value transaction authentication, or account takeovers. Firstly, the identity verification process to set up an account has been fairly well understood, transitioning from in-person, in-branch document checks and document signing to well established online processes involving AI technology and ‘remote’ human checking.
The emerging risk is around account takeovers and high-value transactions, where the potential reward for bad actors can be very high. Therefore the resources applied can be significant. Here, despite layers of security, deepfakes or injection attacks can override current systems, particularly where an account holder’s personal information has been compromised. There is also the growing threat of SIM swaps, where a bad actor can take over a user’s mobile phone account, thereby comprising an additional layer of authentication.
Again, one solution can be to tackle the problem at the source, that is, conduct a face match check at the point of transaction. A biometric map of the user’s face can be stored either by the organisation or the IDSP and taken at the point of account setup. This can then be used to check a user is who they say they are when required. This would also use our anti-spoofing tools of liveness and SICAP to ensure the user is who they say they are and not a presentation or indirect attack, such as replay attacks or injection attacks.
Another quick and simple way of confirming is using a digital ID. For marketplace transactions or high value account withdrawals, the controller can simply request a ‘peer 2 peer’ share from the other party, confirming their name and email address, and within seconds can receive a verified confirmation.