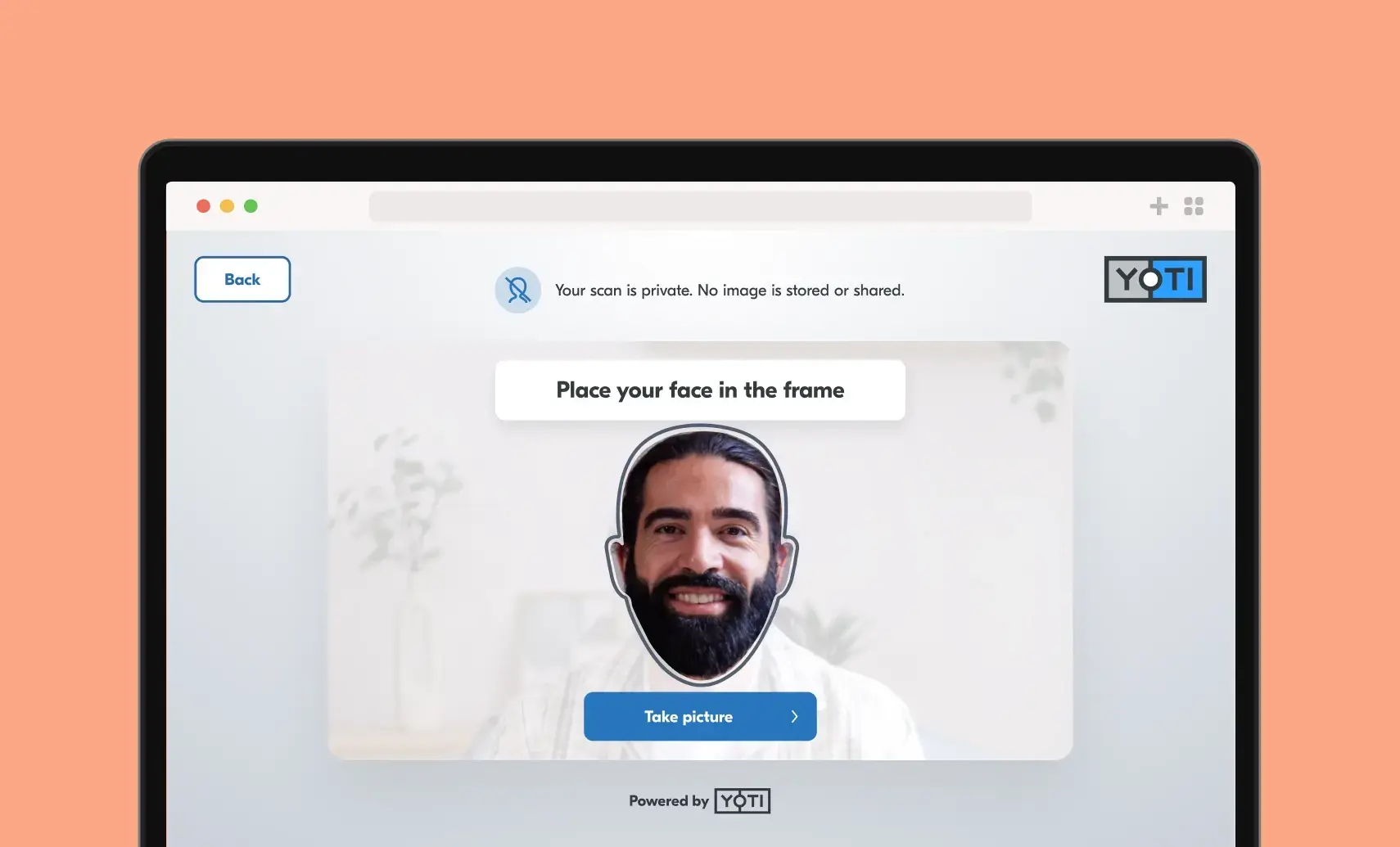
“How accurate is it?” is the first question regulators, businesses and users tend to ask about facial age estimation. To date, we have mainly presented the technology’s Mean Absolute Error (MAE) as a proxy for accuracy. It’s an intuitive way to understand how accurate a model is. We can say it’s accurate to 1.3 MAE for those aged between 13 and 17 years or 2.5 MAE for those aged between 6 and 70 years.
However, the answer is slightly more complicated. Following the COVID-19 pandemic, many people will be more aware of the terms ‘true positive’ and ‘false negative’ in the context of vaccinations and testing, alongside the ‘sensitivity’ of a test. These relate to the level of risk we’re prepared to accept in return for a true result versus a false result.
We can use the same terms when it comes to accuracy of facial age estimation.
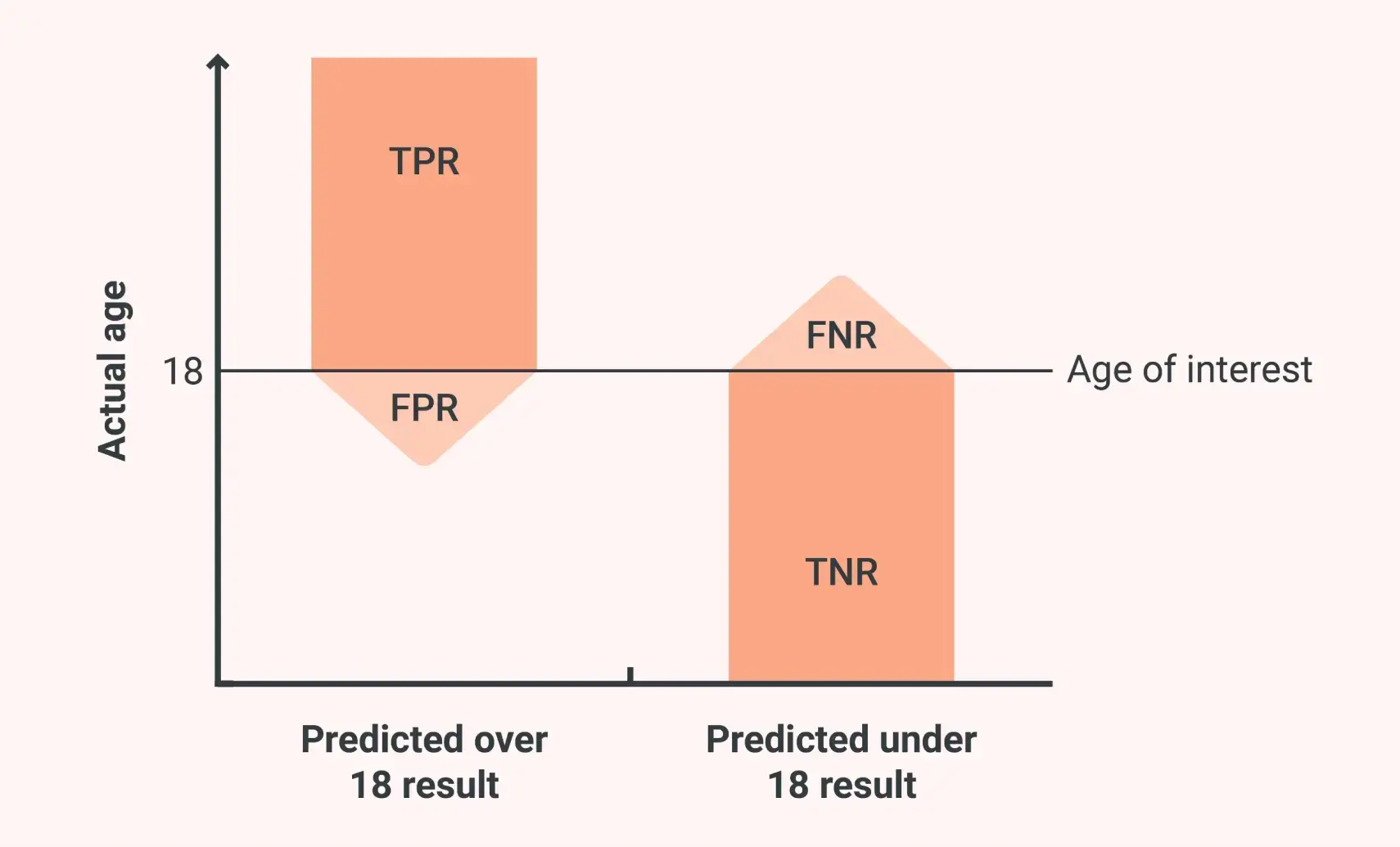
In the graph above, the column on the left shows where a model returns a positive result. In this example, it’s that this person is over the age of 18. The right hand column of graph shows the model returning a negative result – i.e. this person is under 18. Using this example, the following definitions depicted above are:
TPR (True Positive Rate) – a result that correctly estimates the person as over 18
FPR (False Positive Rate) – a result that incorrectly estimates the person as over 18
FNR (False Negative Rate)- a result that incorrectly estimates the person as under 18
TNR (True Negative Rate) – a result that correctly estimates the person as under 18
The goal, of course, is to have the highest possible true negative and true positive (correct or accurate) categories as possible.
Returning to the COVID-19 comparison, false negatives are the worst result. If you had COVID-19, took a test and it came back negative, you were able to go out and potentially spread the virus. That’s our framing of false negatives.
With age, a false negative would occur if a 19-year-old is estimated as under the age of 18. It’s annoying for both the business and customer, but it happens millions of times every day. In contrast, a false positive would occur when a 17-year-old is estimated as over the age of 18. That’s where the main risk is for businesses.
A false negative rate is inconvenient and annoying for customers and organisations, as anyone aged over 18 who has been lucky enough to be asked to show ID will know. But no law is being breached.
A false positive rate is problematic as it can put operators at risk of breaching the law, depending on local regulations.
As such, beyond the ‘accuracy’ question (i.e. the ‘true’ rates), it’s important not to ignore the ‘false’ error rates.
When we talk about accuracy, the false positive rate is the key area of concern. This would result in underaged individuals being incorrectly allowed to access certain age-restricted goods, services or experiences when it is illegal for them to do so. It’s for this reason that we recommend businesses use appropriate safety thresholds.
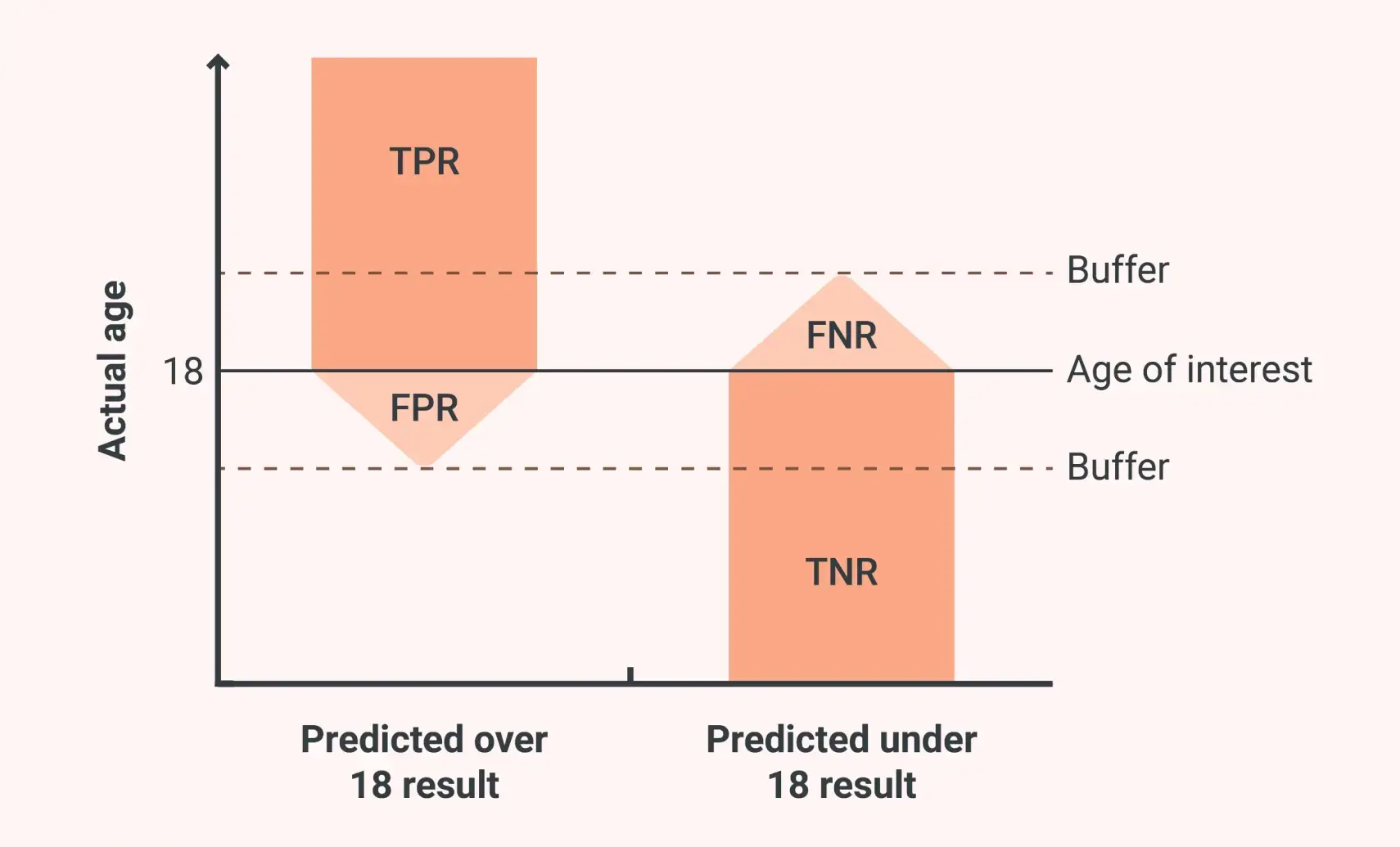
Another common question is, how accurate can facial age estimation get? We continually train our facial age estimation model to improve its accuracy. True positive rates and true negative rates will likely never get to 100%, given the constantly changing differences in people’s lives and circumstances as they age. Although the model is not statistically perfect, it’s still much more accurate than human judgement.
Yet, society has been comfortable with using human age estimation as the accepted method for age verification for decades. In retail settings, we rely exclusively on staff to assess someone’s age visually, within seconds, based on their own life experience and environment.
Now, via initiatives such as NIST evaluation, we can actually measure the accuracy of facial age estimation in a meaningful way using true positive and true negative rates. Given that in most cases, particularly online, there’s no age checking provisions at all, we firmly believe that a scientifically proven, continually improving model is better than not having any measures in place at all.
Our facial age estimation is low cost, scalable, inclusive, privacy-preserving and is already being used globally to create safer, age-appropriate experiences.
To find out more about the accuracy of Yoti’s facial age estimation, you can download our latest white paper.