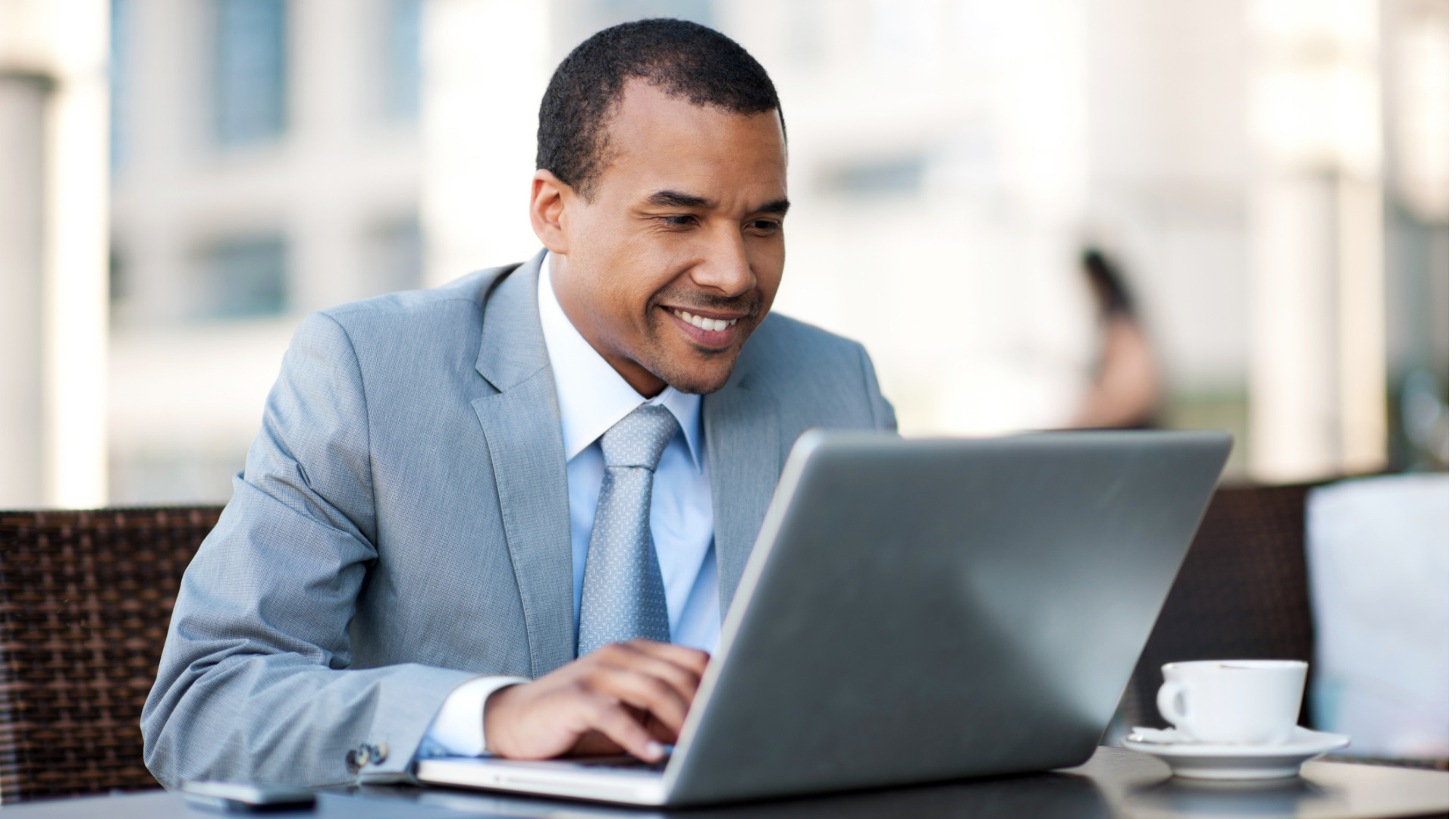
As financial institutions move towards FATF-recommended risk-based AML programs, artificial intelligence (AI) and machine learning can help them be more effective and efficient in the fight against financial crime.
The global framework for fighting financial crime white paper by The Institute of International Finance and Deloitte LLP highlights that: There is growing consensus that the current global framework for fighting financial crime is not as effective as it could be and that more needs to be done at the international, regional and national levels to help identify and stem the flow of illicit finance – an activity which supports some of the worst problems confronting society today, including terrorism, sexual exploitation, modern slavery, wildlife poaching and drug smuggling.
The impact has rippling effects; AML professionals, governments and regulator bodies globally are discussing better approaches to preventing and reducing money laundering from entering financial systems. The most critical area of address is the AML programs institutions must implement within their systems to be compliant.
However, it is challenging for institutions to reform their AML programs. The Money Laundering Bulletin points out that “some of the key problems, and therein some of the most promising solutions, lie with the technology used by companies to underpin their AML programmes. Most of the systems used today originate from the 2000s and were never built for the scale of data or demand we see now.”
In the fight to improve their approaches to identify and reduce money laundering, regulatory bodies and industry leaders acknowledge and encourage the use of Artificial Intelligence (AI) and machine learning to comply with AML regulations. AI and machine learning are proving helpful to implement within anti-money laundering programs to be more effective and meet ever-evolving compliance obligations.
In this article, we will take a look at how AI and machine learning can increase both the efficiency and effectiveness of Anti-money laundering programs.
Why AI and machine learning is important in AML programs
AML programs require compliance teams to gather intensive amounts of customer data as part of the first line of defence during customer due diligence (CDD) processes and ongoing transaction monitoring. This process was previously a manual and repetitive task to analyse if customers present a potential risk.
Here is how AI and machine learning can be applied in different stages of the CDD and ongoing transaction monitoring processes.
AI and machine learning can assist during client onboarding
For AML compliance teams to be compliant, they must carry out Know Your Customer (KYC) processes. They are required to analyse large amounts of data from a range of external sources: adverse information, media, Politically Exposed Persons (PEP), sanctions, and watchlists. To effectively screen an individual, they must gather quality data to assess and know the customers are who they say they are.
Building a better assessment and profile of customers involves being able to match that individual to their identity. It is just as essential to reduce the risk of identity fraud, particularly synthetic identity theft, which is one of the most prominent threats institutions face and is one of the most difficult financial crimes to detect.
Furthermore, Information experts Thomson Reuters explains, “financial institutions often struggle with synthetic identity fraud as well. That’s because fraudsters often open accounts with synthetic IDs to establish credit and behave as stellar customers for months or even years before they use those accounts to defraud financial institutions.”
AI and machine learning can help AML compliance teams verify the identity of an individual in real-time. With biometric face matching, the customer’s identity is scanned and compared with a selfie against a photograph of the customer’s ID. Together both the selfie and ID are assessed in real-time to detect liveness and potential alterations that lead to signs of a fraudulent document.
Ultimately, AI and machine learning aid by speeding up the onboarding experience with a more streamlined onboarding process and provides a more robust first layer in KYC processes to show you have done your CDD checks.
AI and machine learning aids in ongoing transaction monitoring
Money launderers aim to move illicit funds through financial systems as quickly as possible in order to go undetected. These programs are required to conduct continuous monitoring of customers’ transactions to spot potential suspicious behaviour that needs further investigation.
AI can improve the way traditional programs and compliance teams review and source data to build risk profiles on individual customers and improve the recognition of if a customer presents a risk. It scans large data sets at scale, provides categorised breakdowns of profiles, and connects to real-time databases, so compliance officers are alerted as soon as there’s any update in risk status.
In our previous article, regulatory expectations are evolving: how robust is your AML program discussing the challenges slowing progress, we looked at the challenge false positives present. Older programs are known to typically produce false positives that are costly and time-consuming to investigate.
AI can assess while learning from customer data to detect and recognise suspicious behaviour. The ability to better spot any potential risk a customer may present helps AML compliance teams create a more in-depth risk profile. Using machine learning within the transaction monitoring process can increase the scale at which AML compliance teams screen customers’ behaviour and detect risk. The technologies can analyse large quantities of data and further aid compliance officers in ongoing transaction monitoring by more accurately indicating risk.
Additionally, AI has the capability to spot known risks about an individual and make the research process more efficient by improving the time taken. According to the Money Laundering Bulletin, “applying AI to the creation of AML risk data can prevent compliance breaches by spotting previously unknown risks, updating entities faster, identifying remote linkages between entities and enhancing existing profiles with more information (sic) to help make better decisions more rapidly.”
AI and machine learning speed up the process with an analysis of larger sizes of data sets and improved categorised breakdowns of results. AML compliance teams are able to spot suspicious activity quickly, assess the level of threat based on accurate data, review comprehensive customer risk profiles and effectively investigate.
Improve the power of AML professionals decision making
AI and machine learning are not only improving a once static system that struggled to effectively and efficiently screen, onboard and monitor customers. These technologies are helping institutions provide a better customer experience by streamlining the onboarding process and accurately identifying high risk.
With the added benefit of better data, AML compliance teams can make better decisions. They can build a robust risk-based AML program by combining their human expertise with AI and machine learning in the battle of preventing their financial systems from being used for money laundering.
If you would like to learn about how we make compliance simpler, head over to our Identity Verification solution.